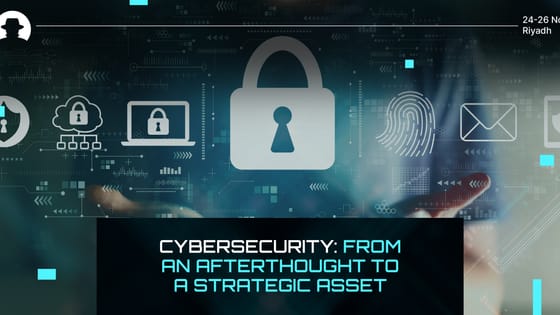
Cybersecurity: From an afterthought to a strategic asset
New research shows that a growing number of organisations view cybersecurity as a strategic priority.
Read MoreInforma Tech’s AI Summit and Black Hat USA recently released a collaborative report entitled How Gen AI Is Revolutionising Threat Detection in Cybersecurity.
It explores major developments in both AI-powered threats, and AI-powered security – because the two are developing side-by-side, each trying to outdo the other and gain an advantage. If cyber criminals gain that advantage, the consequences are potentially devastating; so this is a critical time for cybersecurity professionals and industry leaders to step up to the challenge.
Here, we’ll cover four types of AI threats that are causing disruption around the world right now.
Phishing attacks targeted organisations and individuals around the world with significant success even before GenAI arrived on the scene.
The FBI’s 2023 Internet Crime Report revealed that the total cost of cybercrime in the US rose to $12.5 billion in 2023, with 880,418 complaints logged. A staggering 298,878 (34%) of these were specifically related to phishing.
According to Santander bank, 91% of cyber attacks start with a phishing email.
And with GenAI, phishing can reach more victims with enhanced success rates. In part, this is because the collection and analysis of vast amounts of personal data allow GenAI tools to generate personalised phishing emails that are more effective at deceiving the recipient.
A worldwide survey by iProov in 2023 found that 71% of people globally don’t know what deepfakes are. In spite of this, a surprising 57% said they think they could recognise a deepfake if they saw one.
Deepfake content is on the rise – and it’s highly effective at tricking recipients into believing they’re seeing or hearing a genuine recording.
In 2022, Brazilian crypto exchange BlueBenx also fell victim to a costly deepfake scam – criminals impersonated Patrick Hillmann, the CCO of Binance, and used his likeness on a Zoom call to persuade BlueBenx to send $200,000 and 25 million BNX tokens to their accounts.
AI-powered deepfakes are just happening on Zoom. Scammers have used deepfake YouTube videos to distribute stealer malware (including Raccoon, RedLine, and Vidar); and deepfake audio, often exploiting recordings of the voices of people trusted by victims, is increasingly used to build trust over the telephone.
Threat actors are using AI to generate new malware variants very quickly. They use AI to analyse existing malware code and create slight variants – that are different enough to evade the signature-based detection models used by antivirus software.
Cyber criminals are also using AI to observe and analyse how malware reacts in a sandbox, and use this information to develop detection avoidance techniques in those environments.
There’s growing potential for cyber criminals to manipulate AI-powered systems themselves – turning AI against itself to exploit or harm victims. Vulnerable systems could include autonomous vehicles, chatbots, and critical national infrastructure; so there’s potential for serious harm.
In a recent report titled Adversarial Machine Learning: A Taxonomy and Terminology of Attacks and Mitigations, researchers from the US National Institute of Standards and Technology (NIST) examined four types of attack that must be considered when deploying AI system:
Join us at Black Hat MEA 2024 and discover how to improve your organisation’s cyber resilience.
Join the newsletter to receive the latest updates in your inbox.
New research shows that a growing number of organisations view cybersecurity as a strategic priority.
Read MoreFind out why CISOs and investors are investing in AI-powered integrated cybersecurity platforms.
Read MoreCybersecurity education in schools could empower a new generation of skilled, engaged cybersecurity professionals, and solve the cyber workforce shortage.
Read More