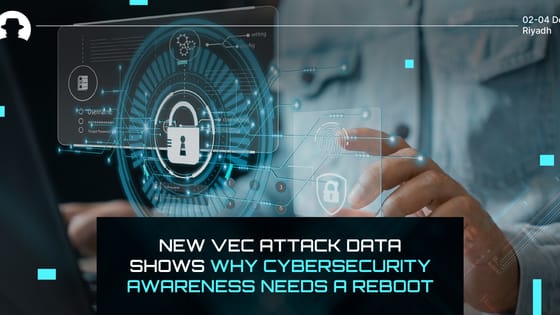
New VEC attack data shows why cybersecurity awareness needs a reboot
New research on vendor email compromise attacks shows that nearly half of employees fall for malicious emails, and 98.5% go unreported.
Read MoreBADBOX is known for selling off-brand connected TV (CTV) and mobile devices via trusted e-commerce retailers and resale sites. The devices are backdoored with an Android malware strain, Triada. And anyone can unknowingly buy a device with pre-installed malware.
Now, researchers at HUMAN Security have linked BADBOX to a new ad fraud botnet called PEACHPIT. At its peak, the botnet infected 121,000 devices per day on Android and 159,000 devices per day on iOS – with PEACHPIT associated apps found in 227 countries and territories.
Those numbers mean that the botnet is powered by millions of hacked devices – and it’s thought that the threat group is working hard to bypass defences.
‘Ad fraud’ refers to any attempt to leverage digital advertising networks for nefarious practices, tricking users into buying or downloading the advertised item for financial gain or to infect the user with malware that can steal sensitive data.
PEACHPIT is an ad fraud branch infecting user devices via a group of 39 apps on Android, iOS, and CTV. Those 39 apps were installed more than 15 million times before they were taken off the market. They’ve affected users in both the private and the public sector, and devices infected with the BADBOX malware enable threat actors to steal data, create residential proxy exit peers, and commit ad fraud for financial gain.
The backdoored devices can also be used to create accounts on WhatsApp or Gmail, by stealing one-time passwords. Because they’re created from a normal smartphone or tablet, these accounts can avoid bot detection.
HUMAN Security is known for disrupting bot attacks and digital fraud. And it has disrupted a core profit-making mechanism of PEACHPIT, working closely with Google and Apple to make it more expensive for attackers to leverage the botnet and remove affected apps from the app stores.
Marion Habiby (Data Scientists at HUMAN) told silicon canals: “The cybercriminals behind PEACHPIT utilised methods such as hidden advertisements, spoofed web traffic, and malvertising to monetise their scheme and defraud the advertising industry. Cybercriminals always follow the money, and our goal at HUMAN is to raise the cost to attackers while lowering the cost to defenders, shorten the window of opportunity for any given threat actor and disrupt the economics of cybercrime.”
It’s a clear example of the reality that ad fraud is becoming increasingly sophisticated. And importantly, it shows that organised crime groups are expanding the scope of their attacks.
BADBOX still operates the deployment of pre-infected devices worldwide, and it’s exploring new avenues (like ad fraud) to reach even more victims at a lower cost. So rather than an ad security issue, this is a much broader security issue – highlighting the need for cybersecurity intelligence to build visibility across a constantly expanding attack surface.
Join the newsletter to receive the latest updates in your inbox.
New research on vendor email compromise attacks shows that nearly half of employees fall for malicious emails, and 98.5% go unreported.
Read MoreWith new data from Arkose Labs, learn why psychology, business strategy and timing are just as important as technology for cyber defence.
Read MoreThe latest in a long line of attacks against IoT devices that highlights the vulnerability of the Internet of Things.
Read More